ID-Booth: Identity-consistent Face Generation with Diffusion Models
Darian Tomašević 1, Fadi Boutros 2, Chenhao Lin 3, Naser Damer 2,4, Vitomir Štruc 5, Peter Peer 1
Poster presentation at FG 2025
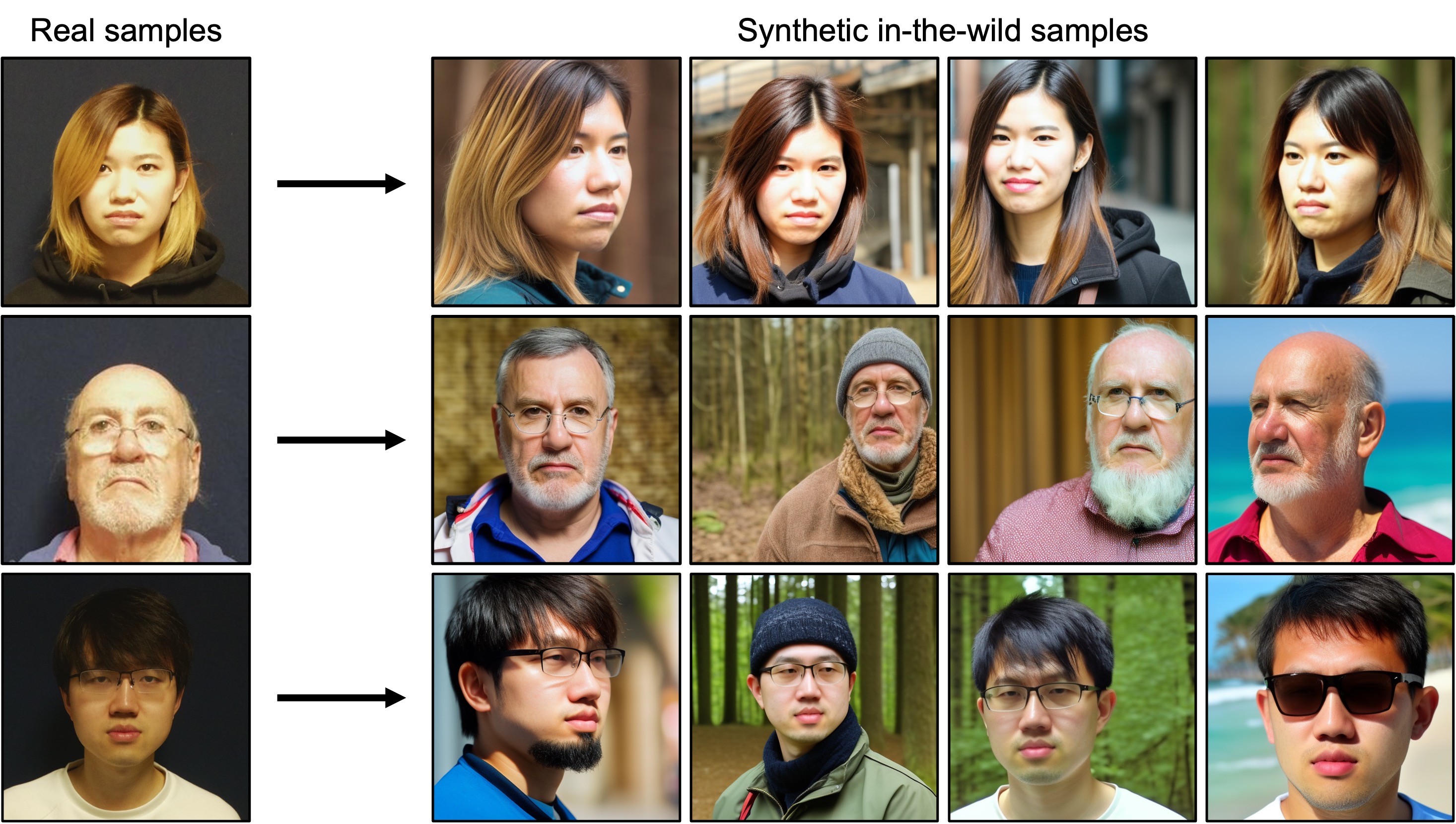
We introduce the ID-Booth framework, which:
🔥 generates in-the-wild images of consenting identities captured in a constrained environment
🔥 uses a triplet identity loss to fine-tune Stable Diffusion for identity-consistent yet diverse image generation
🔥 can augment small-scale datasets to improve their suitability for training face recognition models
Abstract
Recent advances in generative modeling have enabled the generation of high-quality synthetic data that is applicable in a variety of domains, including face recognition. Here, state-of-the-art generative models typically rely on conditioning and fine-tuning of powerful pretrained diffusion models to facilitate the synthesis of realistic images of a desired identity. Yet, these models often do not consider the identity of subjects during training, leading to poor consistency between generated and intended identities. In contrast, methods that employ identity-based training objectives tend to overfit on various aspects of the identity, and in turn, lower the diversity of images that can be generated. To address these issues, we present in this paper a novel generative diffusion-based framework, called ID-Booth. ID-Booth consists of a denoising network responsible for data generation, a variational auto-encoder for mapping images to and from a lower-dimensional latent space and a text encoder that allows for prompt-based control over the generation procedure. The framework utilizes a novel triplet identity training objective and enables identity-consistent image generation while retaining the synthesis capabilities of pretrained diffusion models. Experiments with a state-of-the-art latent diffusion model and diverse prompts reveal that our method facilitates better intra-identity consistency and inter-identity separability than competing methods, while achieving higher image diversity. The produced data allows for effective augmentation of small-scale datasets and training of better-performing recognition models in a privacy-preserving manner.
Overview
To fine-tune a pretrained Stable Diffusion model on a specific identity, the ID-Booth framework utilizes three training objectives: reconstruction loss, prior preservation loss, and the novel triplet identity loss. The first two are aimed at the reconstruction of training and prior images, respectively. The latter focuses on the identity similarity between generated samples and both training and prior samples to improve identity consistency without impacting the capabilities of the pretrained model.
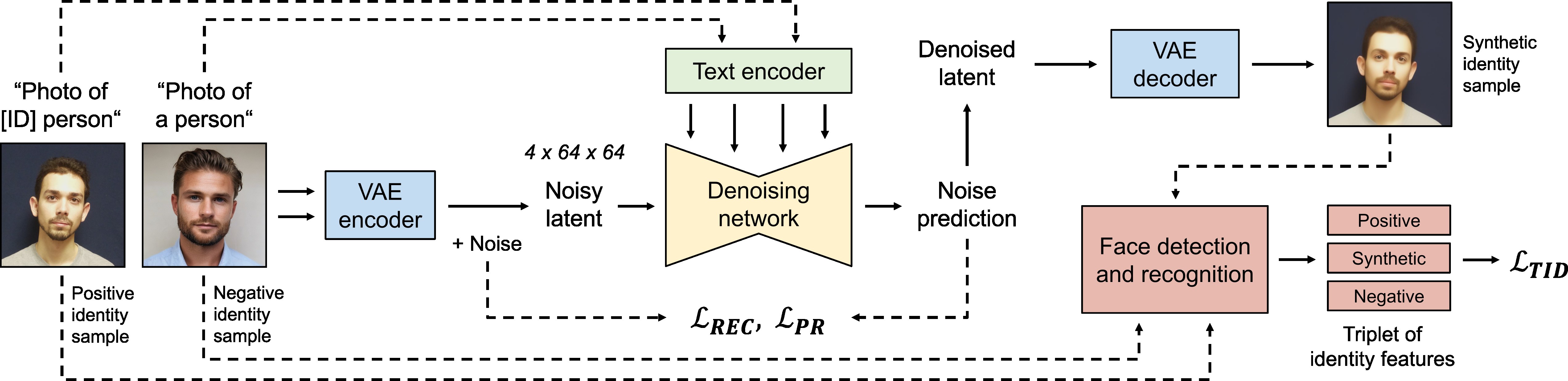
Comparison with existing methods
The proposed ID-Booth framework achieves better identity consistency than DreamBooth and better image diversity than PortraitBooth. In turn, it enables better augmentation of small-scale lab-setting recognition datasets.
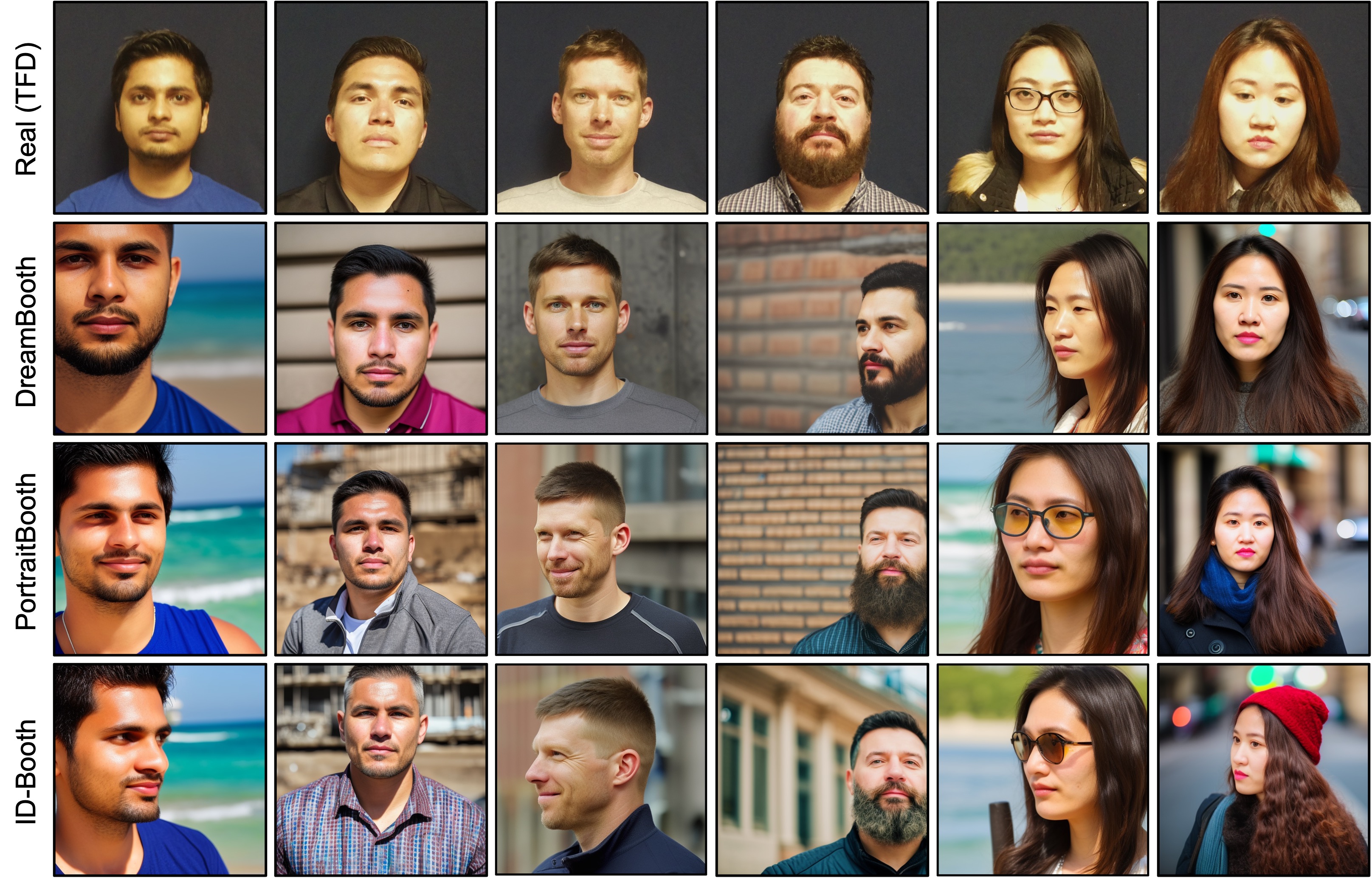
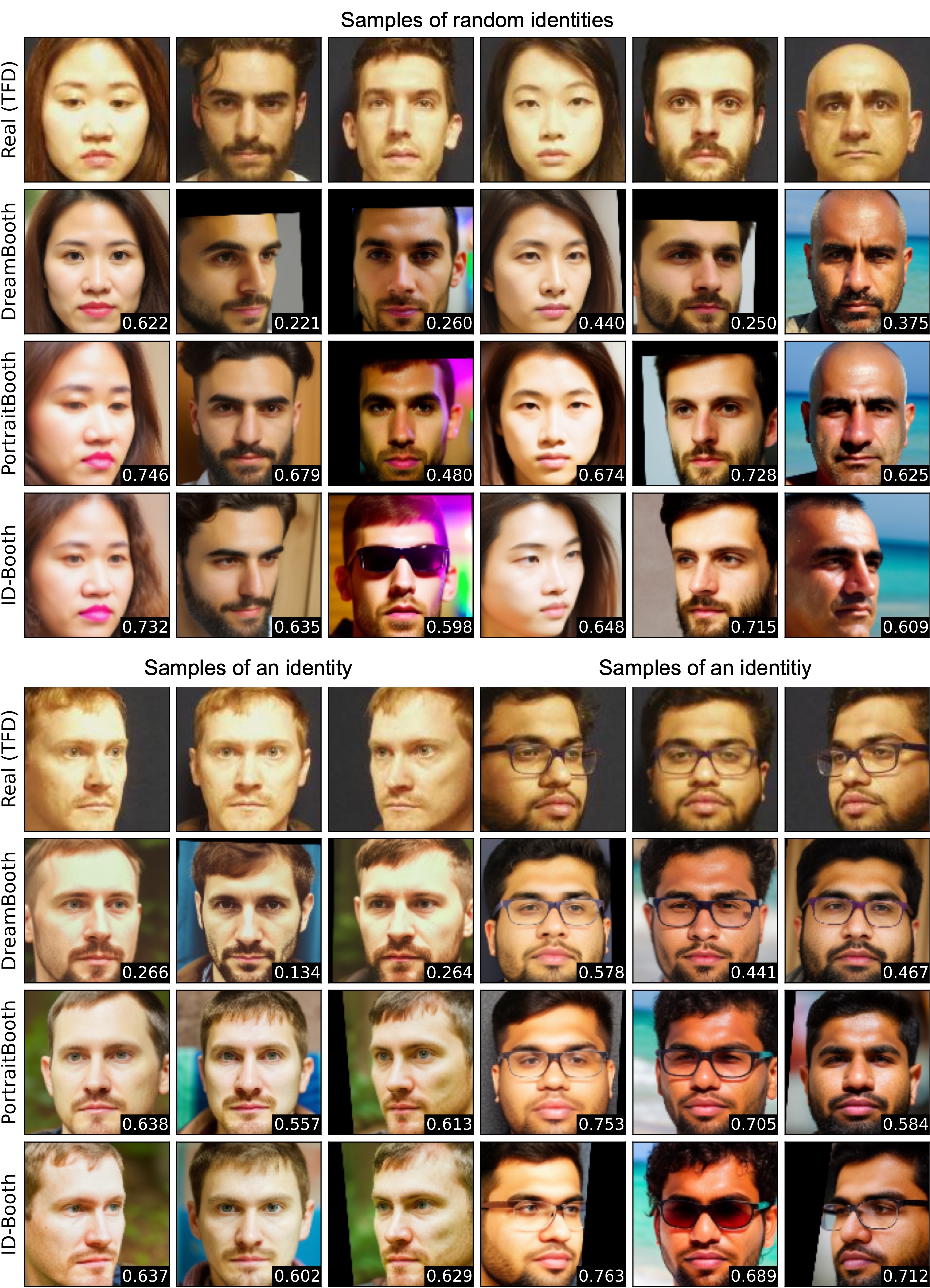
BibTex
@article{tomasevic2025IDBooth,
title={ID-Booth: Identity-consistent Face Generation with Diffusion Models},
author={Toma{\v{s}}evi{\'c}, Darian and Boutros, Fadi and Lin, Chenhao and Damer, Naser and {\v{S}}truc, Vitomir and Peer, Peter},
journal={arXiv preprint arXiv:2504.07392},
year={2025}
}